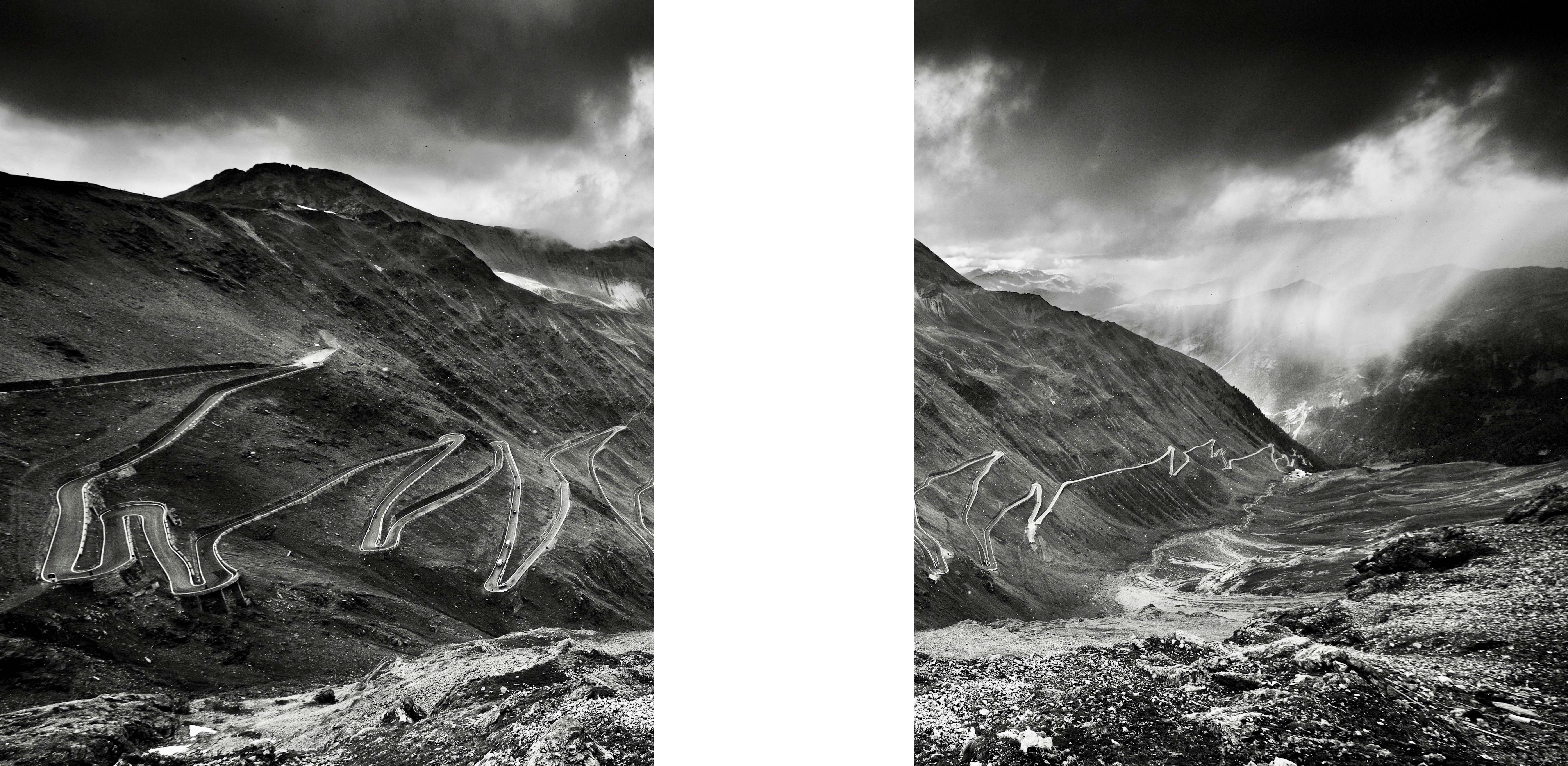
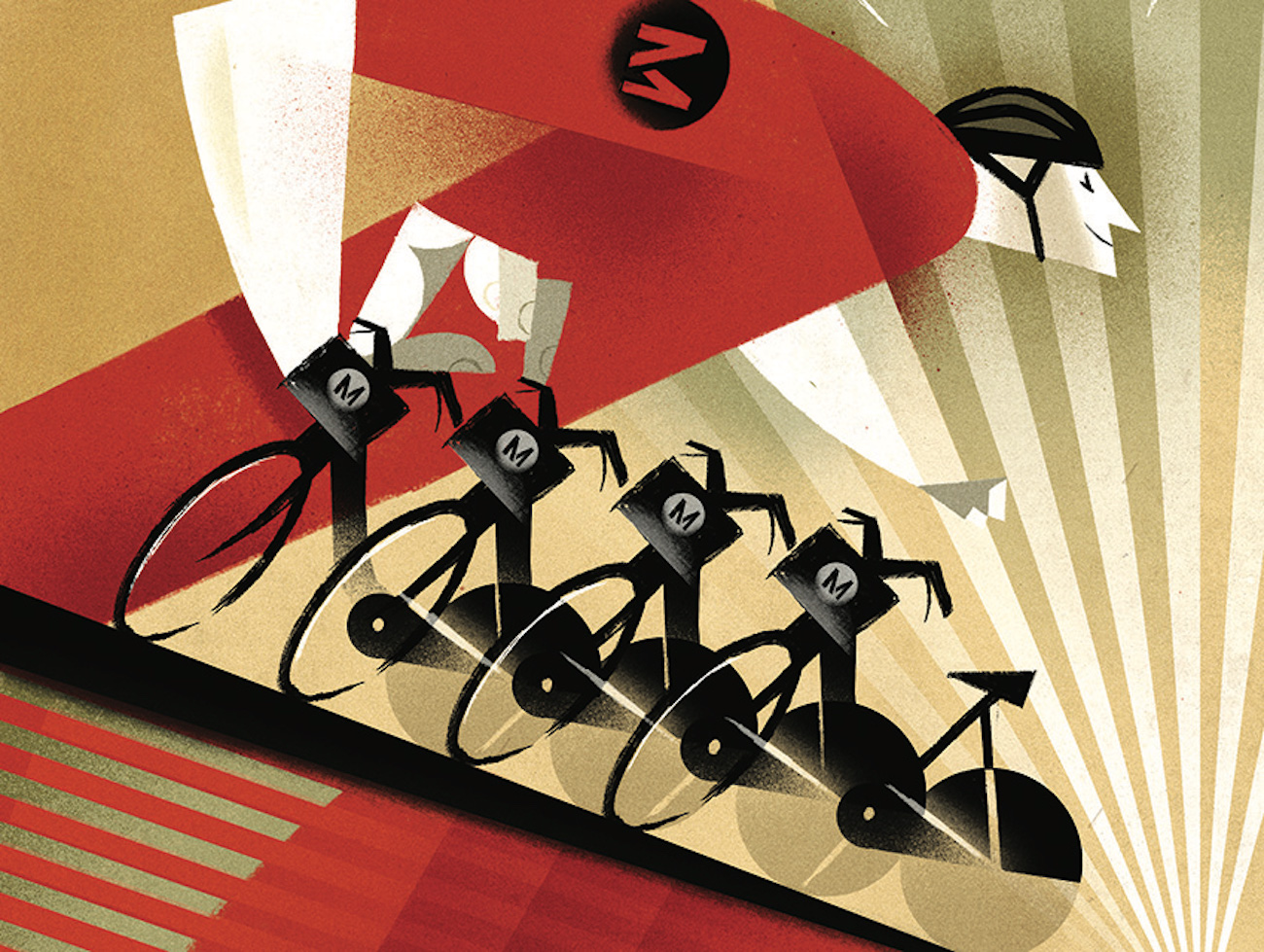
According to a new study, even a relatively short walk to find the nearest bike is enough to deter many people from using a bike-share system.
“If a docking station is more than two or three blocks away, they just won’t go there,” said Karan Girotra, professor of operations, technology and innovation at Cornell Tech and the Cornell SC Johnson College of Business. “And if they encounter a station without bikes, it’s very unlikely they will go to the next station.”
Girotra co-authored “Bike-Share Systems: Accessibility and Availability,” published in November by Management Science, with Elena Belavina, associate professor at the School of Hotel Administration in the SC Johnson College, and Ashish Kabra, assistant professor at the University of Maryland’s Robert H. Smith School of Business.
Their findings imply that, outside of a few big stations at major transit hubs, cities and bike-share operators should strive to create denser networks with many smaller stations, Girotra and Belavina said, and keep them stocked.
“It’s no surprise that people want stations close to them, but it’s much closer than most planners and bike-share systems thought they needed,” Belavina said. “Most systems are nowhere close to their optimal density.”
Bike sharing systems hold the potential to reduce traffic and pollution in dense, flat cities such as London, New York, Paris and Shanghai, the researchers noted. They encourage and enhance public transit use by providing “last mile” connections to bus and train stations.
But “their promise of urban transformation is far from being fully realized,” according to the paper. Many systems were established quickly, sometimes through public-private partnerships, and with less rigorous planning than higher-cost transit systems, Girotra said.
“There was perhaps an opportunity to put a little more thought into how a bike-share system can be introduced in a city,” he said.
To that end, the research team built a model to produce the first estimates of how station proximity and bike availability influence bike-share operations.
The structural demand model analyzed data from Paris’ Vélib’ system — the largest outside China with roughly 17,000 bikes and 950 stations — during four months of 2013, a period that included nearly 4.4 million trips. The data provided snapshots of system usage every two minutes, showing how stations changed throughout each day.
The researchers blended that information with data about population density in different city districts, metro ridership, attendance at top tourist destinations and weather conditions. The team also logged the locations of thousands of points of interest such as transit stations, parks, libraries, hotels, grocery stores, restaurants and cafes.
“Put together,” Belavina said, “that gave us some ability to disentangle what guides people’s decisions in choosing bike sharing and different bike-share stations.”
The model determined that someone roughly 300 meters (nearly 1,000 feet) from a docking station is 60% less likely to use the service than someone very near the station. The odds decrease slightly with every additional meter, such that someone 500 meters away — about one-third of a mile — is “highly unlikely to use the system.”
But a 10% increase in bike availability — the likelihood of finding a bicycle at a station — would grow ridership by roughly 12%, the study found, thanks to fewer lost sales at out-of-stock stations and improved expectations of the system.
Among the various points of interest, placing stations near grocery stores provides the most benefit, the model showed.
Generating the study’s findings required methodological advances to adapt demand modeling to a bike-share context, the researchers said.
Models have long predicted shifts in usage patterns when considering new locations for transit stations, retail outlets or bank ATMs. But bike-share demand in a major city, with hundreds of stations changing inventory throughout each day, involved studying a more dynamic system with much finer resolution, Girotra said.
The team’s huge volume of data might have required completing more than a quadrillion calculations to generate the best estimates, likely taking over a year, according to the paper. Instead, the researchers developed new computational techniques, Belavina said, to condense some data and make the process more manageable.
The resulting model, according to the co-authors, can be applied not only to bike-share systems but other micro-mobility services: scooters, powered bikes, local food delivery and ride-sharing. The researchers plan to look more broadly at micro-mobility in a future study partnering with London’s transit agency.
Regarding bike sharing, the study’s advice was clear: “Make bikes and stations more available,” Girotra said. “People don’t like walking to access a bike-share system.”